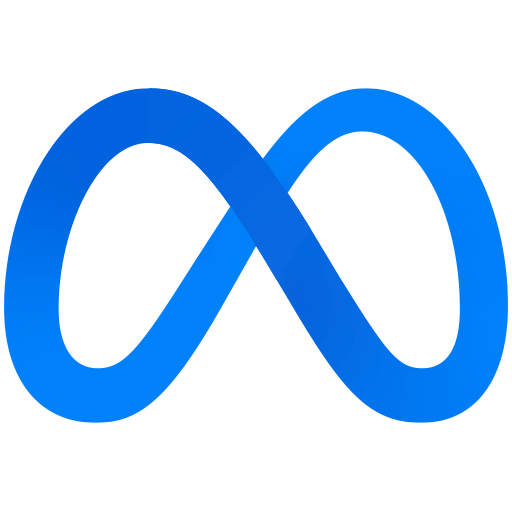
Meta I-JEPA stands for Meta Integrated Joint Environmental Protection Agreement. It is a comprehensive environmental agreement designed to promote environmental protection and sustainable development. This agreement focuses on addressing various environmental issues through collaborative efforts between different entities or countries. Meta I-JEPA aims to establish standards and guidelines for environmental protection, encourage the adoption of eco-friendly practices, and support the implementation of sustainable development initiatives. By fostering cooperation and coordination in environmental conservation efforts, Meta I-JEPA seeks to contribute to a healthier and more sustainable planet for current and future generations.
Meta I-JEPA was created by Yann LeCun, Meta's Chief AI Scientist. LeCun proposed a new architecture focused on developing machines capable of learning internal models of the world to enhance learning speed, task planning, and adaptability. I-JEPA, the first AI model based on this vision, utilizes abstract representations of images for learning, leading to strong performance in computer vision tasks with increased computational efficiency. The model's representations are versatile across applications without extensive fine-tuning.
To use Meta I-JEPA, follow these steps:
Objective: The aim is to assign high energy to incompatible inputs and low energy to compatible inputs using Joint-Embedding (invariant) Architectures, Generative Architectures, and Joint-Embedding Predictive Architectures.
Core Concept: I-JEPA focuses on predicting missing information in an abstract representation to enhance semantic features and uses a multi-block masking strategy for producing semantic representations.
Architecture Overview: I-JEPA utilizes a Vision Transformer (ViT) as a context encoder and a narrow ViT as a predictor to generate representations of target blocks within an image.
Efficiency and Performance: I-JEPA demonstrates computational efficiency by processing only one view of an image by the target encoder and context blocks by the context encoder. It outperforms pixel and token-reconstruction methods on tasks like ImageNet-1K linear probing and semi-supervised evaluation.
Training and Evaluation: The model efficiently learns off-the-shelf semantic representations without hand-crafted view augmentations and competes well with prior pretraining approaches, particularly excelling in object counting and depth prediction tasks.
Visualization and Interpretation: I-JEPA's strong performance is evidenced by correctly capturing positional uncertainty and producing high-level object parts accurately without discarding positional information.
By following these steps, users can effectively utilize Meta I-JEPA for tasks that require semantic representation learning and efficient computational processing.
No reviews found!