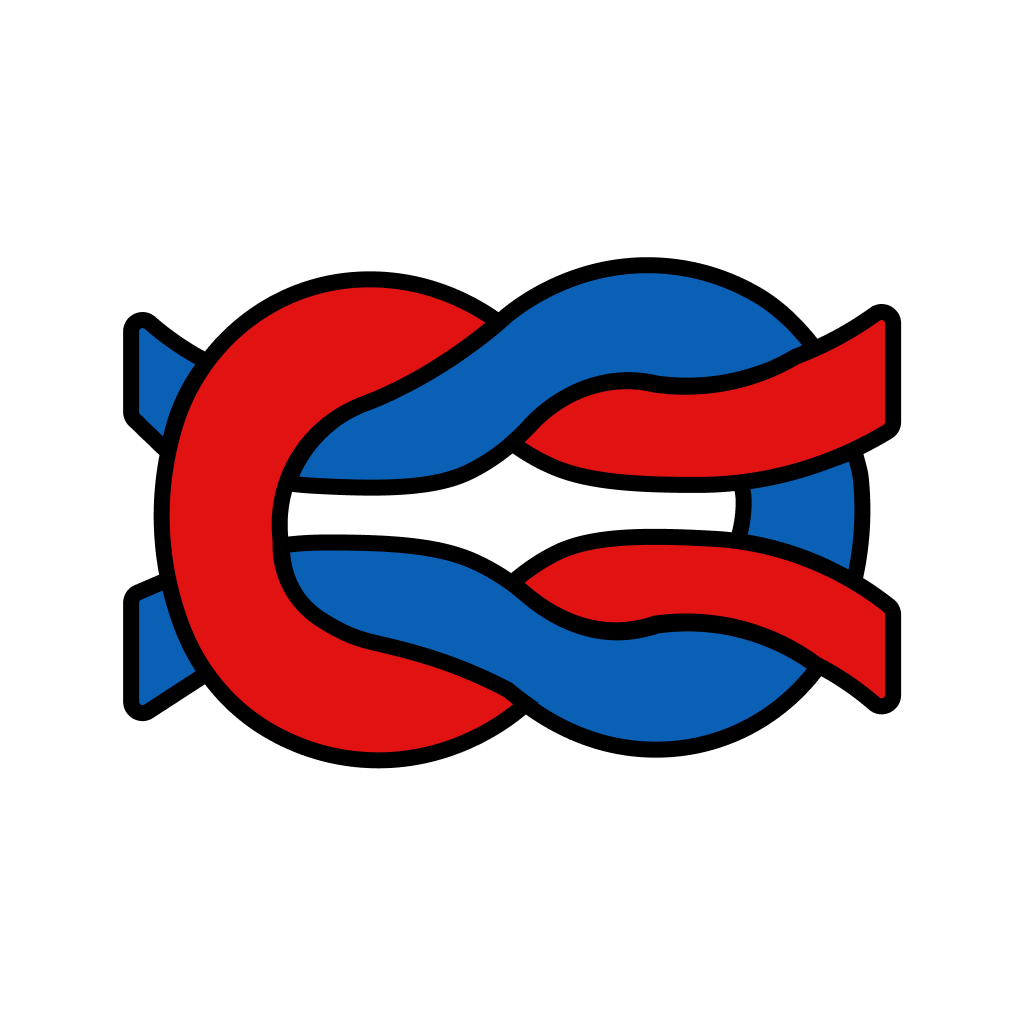
Langfuse is an open-source LLM Engineering Platform that provides observability, metrics, evaluations, prompt management, and a playground for debugging and improving LLM apps. It is designed to be compatible with any model or framework, allowing for complex nesting and offering open APIs for building downstream use cases. Langfuse has been positively reviewed for its effectiveness in monitoring GPT usage, analyzing LLM applications, and providing detailed latency and analytics in the market. Users have praised it for its ease of integration, intuitive usage, and fast iterations by the development team. Langfuse is known for its security features, being SOC 2 Type II and ISO 27001 certified, GDPR compliant, and built with security in mind.
Langfuse was created by a team comprising Clemens, Marc, and Max. The company provides an open-source LLM Engineering Platform for observability and analytics in LLM apps. Langfuse is geared towards improving costs, latency, and response quality using intuitive dashboards and tools for monitoring GPT usage and debugging LLM applications.
To use Langfuse, follow these steps:
Sign Up: Create an account on Langfuse's platform by visiting their website and registering for an account.
Choose a Tier: Select the appropriate tier based on your needs. Langfuse offers Hobby, Pro, and Team tiers, each with different features and usage limits.
Access Features: Explore the platform's features such as observability, tracing, prompt management, and metrics to debug and enhance LLM (Large Language Model) apps.
Integrate with LLM Apps: Langfuse works with any model or framework. You can use it to monitor GPT usage, token usage, monitor hallucinations, and trace request history.
Utilize Collaboration Tools: Depending on your tier, you can collaborate with team members on projects, access unlimited data, and enjoy different levels of support like community support or dedicated support.
Ensure Security and Compliance: Langfuse offers security features like SSO (Single Sign-On), role-based access control, and compliance with data processing agreements like GDPR.
Review Documentation: Refer to Langfuse's documentation available on their website and GitHub repository for detailed information on using the tool.
Stay Updated: Keep track of any recent launches, reviews, and updates related to Langfuse to make the most of the platform's capabilities and features.
Langfuse provides a valuable resource for monitoring and improving the performance of LLM applications. By following these steps, users can effectively leverage Langfuse's features and enhance their LLM projects efficiently.
I appreciate the ease of integration with various LLM models and frameworks. The open APIs allow me to build downstream use cases efficiently, which has significantly streamlined our development process.
While the tool is generally intuitive, I found the documentation to be a bit lacking in certain areas, especially for more advanced features. It could definitely use some improvements.
Langfuse addresses the need for observability in LLM applications effectively. By providing detailed latency metrics and analytics, I can identify bottlenecks in our models, which leads to faster iterations and improved performance.
The security features are impressive, especially the SOC 2 Type II and ISO 27001 certifications. Knowing that our data is secure gives us peace of mind as we work on sensitive projects.
I wish the playground for debugging was a bit more user-friendly. Sometimes it can feel overwhelming with all the options available.
Langfuse allows me to monitor our GPT usage closely, which helps in optimizing our resources and improving response times in our applications. This directly benefits our user experience.
The prompt management feature is fantastic! It allows me to test and iterate on prompts easily, leading to better outcomes in our LLM applications.
The initial setup took some time, but once I got through that, everything else was smooth sailing.
Langfuse has helped us with real-time monitoring of our LLM apps. This has resulted in quicker debugging and better maintenance, which is crucial for our deployment times.