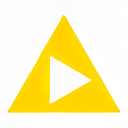
KNIME is a free and open-source data analytics, reporting, and integration platform that integrates various components for machine learning and data mining through its modular data pipelining concept. It offers a complete platform for end-to-end data science, allowing users to create analytic models, deploy them, and share insights within the organization through data apps and services. The KNIME Analytics Platform is an open-source software with a visual interface that enables users to build analyses of any complexity level without coding, from automating spreadsheets to ETL to machine learning. Users can access, blend, analyze, and visualize data, as well as integrate their favorite tools and libraries as needed.
KNIME was co-founded by Michael Berthold, Sven Dorn, and Christian Dietz in 2008. The company aims to provide an open-source data analytics, reporting, and integration platform. KNIME integrates various components for machine learning and data mining through a modular data pipelining concept. The platform allows users to build analyses of any complexity level without coding, making it accessible and intuitive for data analysis tasks.
To use Knime, follow these steps:
KNIME Analytics Platform: This open-source software offers a visual interface for building analyses of various complexity levels, from automating spreadsheets to machine learning. Users can access, blend, analyze, and visualize data without coding, and integrate additional tools and libraries as needed.
KNIME Hub: This commercial software enables collaboration and scaling of data science. It can be accessed online as KNIME Community Hub or installed into a company's private infrastructure as KNIME Business Hub. It provides a unified environment for users of different expertise levels to work together.
KNIME Community Hub: Users can explore and learn from numerous working examples of data science solutions on this platform. It allows users to benefit from community contributions, upskill, and delve deeper into the field of data science. Small groups can also collaborate on solutions in private spaces.
KNIME Business Hub: This platform facilitates collaboration and sharing within a company's dedicated infrastructure. Teams can publicly share knowledge across the organization or privately within the team. With robust productionization capabilities, data experts can deploy and monitor workflows, share them as data apps and services, and ensure data-driven decision-making across the enterprise.
By following these steps, users can leverage Knime to its full potential for various data analytics, reporting, and integration needs.
No reviews found!