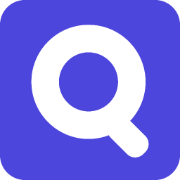
QuarkIQL was a tool designed to simplify the testing of Computer Vision APIs by enabling users to create custom test images easily. It leveraged powerful image diffusion models to streamline the process of generating images through text prompts. Although innovative, QuarkIQL is no longer available for use. Its key features included the ability to support various types of API requests like GET and POST, aimed at accelerating development goals. Additionally, QuarkIQL kept a historical log of queries to help developers conduct extensive experiments without losing progress and was developed by a team of software engineers with academic backgrounds in engineering and operations research.
QuarkIQL was created by two software engineers: Kevin Yu, who holds a B.S. in Mechanical Engineering from the University of Texas at Austin, and Jake Wigal, who has an M.S. in Operations Research from the Naval Postgraduate School. The platform aimed to facilitate Computer Vision API testing by enabling users to generate custom test images easily using image diffusion models.
QuarkIQL was a versatile tool for simplifying Computer Vision API testing by enabling users to generate custom test images effortlessly. The tool's key features included access to powerful image diffusion models that could create test images based on text prompts. However, it is important to note that QuarkIQL is no longer available for public use. Users could utilize QuarkIQL to make a variety of requests such as GET and POST, allowing them to progress efficiently towards their development objectives. Despite its innovative functionalities, QuarkIQL has been discontinued, marking the end of its support and availability for users.
The image quality was often outstanding, and I could generate exactly what I needed for our API tests.
The user interface could have been more intuitive, as I found it slightly confusing at first.
QuarkIQL solved the problem of sourcing test images, allowing us to create exactly what we needed quickly and efficiently, which improved our testing framework.
The concept of generating images from text prompts is innovative and very useful for testing purposes.
The performance was inconsistent at times; I experienced some lag when generating images, which was frustrating during urgent testing phases.
It reduced the time spent on image sourcing, but the performance issues sometimes negated those benefits.
The custom image generation was a game-changer for our testing processes. I could create precisely what I needed without searching for stock images.
The initial setup was a bit complicated, especially for those not familiar with API testing.
It helped bridge the gap between development and testing, allowing my team to focus on refining our algorithms without worrying about image sourcing.