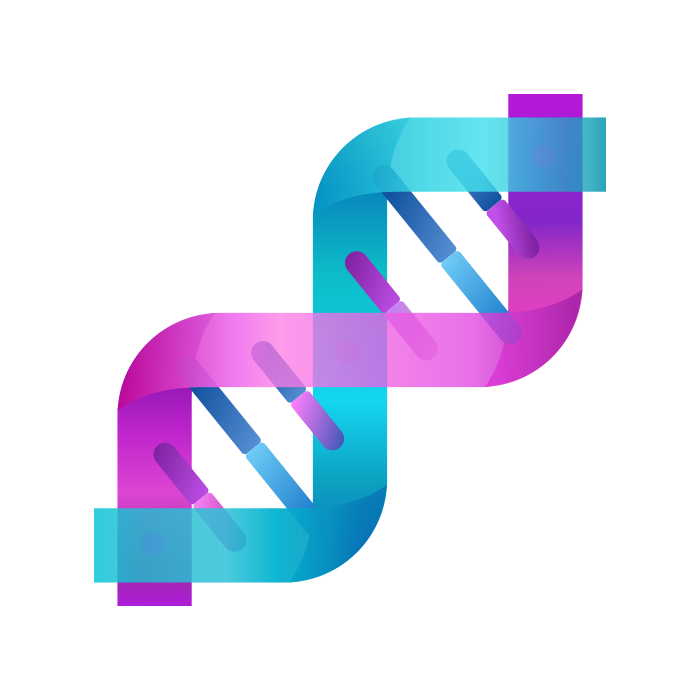
Dstack is an open-source orchestration engine that simplifies developing, training, and deploying AI models while managing clusters on any cloud or data center. It provides a user-friendly interface for AI engineers to seamlessly work on development, training, and deployment tasks without depending on additional tools or support from the Ops team. Dstack also supports multiple cloud providers and offers features tailored for AI development, making it more lightweight and specialized compared to Kubernetes. Moreover, it enables easy integration with new cloud providers and allows for the efficient utilization of on-prem servers by automatically managing them as a fleet for running containers.
The creator of Dstack and detailed company information is not available in the documents provided. If you have any other questions or need assistance with different topics, feel free to ask!
To use Dstack effectively, follow these steps:
Get Started Quickly: Dstack is open-source and self-hosted, allowing you to use your cloud accounts or data centers like AWS, Azure, GCP, and others. Sign up and start in minutes.
Utilize Multiple Cloud Providers: You can leverage various cloud providers or on-prem infrastructure with Dstack, using any hardware and open-source frameworks for both training and deployment.
Development Environments: Set up remote machines with your code and favorite IDE easily using dev environments. This enables interactive coding before deploying models or scheduling tasks.
Scheduling Tasks: Easily schedule batch jobs like training, fine-tuning, or data processing using tasks. Run tasks on a single machine or a cluster of nodes based on your requirements.
Deploy Models: Services in Dstack make deploying models as endpoints simple, allowing you to create secure, public, and scalable endpoints effortlessly.
Manage Clusters with Fleets: Use fleets to efficiently provision and manage clusters and instances, both on the cloud and on-premises. Fleets can be reused for dev environments, tasks, and services.
Consider Dstack Sky: If you prefer not to host the Dstack server yourself or need access to GPUs through a marketplace, sign up for Dstack Sky. It offers hosted servers and access to cloud GPUs at competitive rates.
By following these steps, you can make the most of Dstack for developing, training, and deploying AI models while managing clusters across various cloud platforms or data centers.
I appreciate the open-source aspect of Dstack. It's nice to have a tool that is customizable and free to modify as needed.
The documentation can be a bit lacking at times. I often find myself searching for specific examples to understand how to implement certain features.
Dstack helps streamline the deployment process of AI models. This saves me time, but sometimes I wish it had more robust integrations with other tools.
The user interface is very intuitive. It makes managing AI models much easier compared to other orchestration tools I've used.
Sometimes the performance can lag when deploying larger models, which can be frustrating.
Dstack provides a unified platform for model training and deployment, which significantly reduces the friction in our workflow.
I love how Dstack simplifies the process of managing clusters. It's particularly great for teams that lack extensive DevOps support.
There are times when I wish it had more advanced features for monitoring and analytics.
Dstack allows us to efficiently utilize our on-prem servers, which helps reduce costs and makes our operations much smoother.