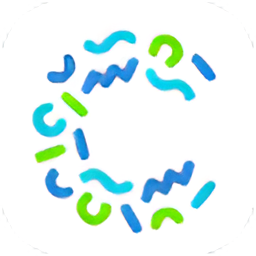
Cradle Bio is a groundbreaking AI tool designed to assist biologists in enhancing protein design. By utilizing advanced prediction algorithms and AI design recommendations, Cradle Bio enables biologists to streamline the visualization, analysis, and prediction of potential protein candidates, ultimately saving time and improving the efficiency of protein design processes. It is equipped to handle multiple properties and optimization tasks simultaneously, learning from each round of experimentation to provide better variants and significantly reduce time-to-market. Additionally, Cradle Bio ensures data privacy, security, and full intellectual property ownership for users.
Cradle was founded by Sylvain Gariel, who currently serves as the Chief Operating Officer (COO) at DNA Script. The company is driven by a team that combines expertise in biology, design, engineering, and machine learning, with members who have previously contributed to products and technologies at notable companies like Google, Deepmind, and Uber. Cradle operates from locations in Zurich, Switzerland, and Amsterdam, Netherlands, hosting regular team gatherings to collaborate on tackling significant challenges in machine learning and biology.
To use Cradle, follow these steps:
Sign Up and Log In: Create an account on Cradle's platform and log in to access the tool.
Explore Features: Familiarize yourself with Cradle's top features like powerful prediction algorithms, AI design suggestions, and the ability to visualize, analyze, and predict candidates' potential in one place.
Design Proteins: Start designing proteins by inputting your sequences and exploring the AI design suggestions provided by Cradle.
Iterate and Improve: Cradle learns from each round of experimentation, offering better variants and speeding up the design process. Continuously test and iterate to enhance protein designs.
Handle Multiple Properties: Unlike traditional methods, Cradle is designed to handle multiple properties and optimization tasks in a single round, making it efficient and effective.
Ensure Data Security: Rest assured that your sequences and data are kept private and secure with Cradle, ensuring full ownership of intellectual property.
Benefit from Continuous Improvement: By training the AI with results from lab experiments, Cradle continuously enhances candidates with each testing round, leading to better results over time.
Save Time and Increase Productivity: Utilize Cradle to reach objectives in half the time, thanks to its learning capabilities and efficient design tools.
These steps will help you effectively use Cradle to design improved proteins, optimize thermostability, predict protein structures, and achieve your protein design goals efficiently and securely.
File Source: Cradle User Manual.
No reviews found!