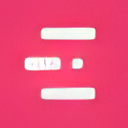
The ability to select from various GPU options from different cloud providers gives me flexibility depending on my project needs.
I noticed that the autoscaling feature can be a bit slow to respond during peak loads.
It allows for efficient resource allocation, keeping costs low while delivering high performance in ML tasks.
I appreciate the pay-per-use pricing model which allows me to manage costs effectively while scaling my machine learning applications.
The initial setup took some time to understand, especially for someone new to cloud computing.
Cerebrium AI helps me optimize my ML workflows, reducing latency significantly and improving responsiveness in real-time applications.
The platform has a lot of potential with its features.
Unfortunately, I experienced some significant latency issues that affected my application performance.
While it promises high reliability, my experience has shown inconsistent performance, which can be a dealbreaker.
The autoscaling feature is convenient for handling varying workloads.
I had some trouble with the setup process, which was not as straightforward as I expected.
It helps manage resources efficiently, but the initial confusion can be challenging for new users.
The high reliability with 99.999% uptime is impressive and crucial for my applications.
There are some features that I think could be more intuitive for new users.
It simplifies the deployment of my ML applications, allowing me to focus on data rather than infrastructure management.
The service is affordable for small projects, which is great for independent developers like me.
The interface could use some modernization; it feels a bit dated.
It allows me to experiment with different ML models without committing to expensive infrastructure upfront.
The variety of GPU options is impressive and allows for tailored solutions.
I encountered some bugs that disrupted my workflow, which was frustrating.
It aims to provide a flexible computing environment, yet the execution can be hit or miss.
The low latency feature has been great for my real-time models, allowing them to perform efficiently.
The documentation is lacking in certain areas, making troubleshooting a bit challenging.
It allows me to scale my ML applications without the upfront costs, which is beneficial for small projects.
The observability tools are helpful in analyzing application performance.
I found the pricing structure to be somewhat confusing, especially for new users.
It assists in managing computing resources, but I feel there are more user-friendly alternatives.
I like the cost management tracking feature; it helps me keep detailed records of my spending.
Customer support can be slow to respond, which is frustrating when I have urgent issues.
It enables me to run complex ML models without needing extensive infrastructure, which saves both time and money.
The real-time logging feature is incredibly useful. It helps me monitor my applications' performance on the fly.
Sometimes the interface feels a bit overwhelming due to the variety of options available.
Cerebrium AI reduces the complexity of scaling ML applications, allowing me to focus more on building algorithms rather than managing infrastructure.