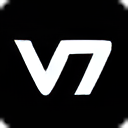
The ability to create customized evaluation suites is a major advantage in our testing process.
The documentation could be improved, especially regarding advanced features.
It helps us maintain high-quality outputs from our models, which is essential for user trust.
The comprehensive evaluation options provided by BenchLLM allow us to thoroughly assess our models before deployment.
It can be resource-heavy on our system, especially during large-scale testing.
It enables us to catch issues early in the development cycle, preventing costly mistakes later on.
The detailed quality reports are incredibly useful for our team. They provide insights that help us make informed decisions about model adjustments.
I would like to see more examples in the documentation to help new users get started faster.
It aids us in monitoring the performance of our models in real-time, which is essential for maintaining high standards in our applications.
The interface is user-friendly once you're familiar with it, and it integrates seamlessly with our CI/CD processes.
The initial learning phase can be a bit challenging, especially for team members new to CLI tools.
It helps us streamline the testing process, ensuring that we catch any regressions before they reach production.
The testing framework is robust and really customizable, which is crucial for our diverse projects.
The system requirements can be quite demanding, especially for larger-scale evaluations.
It helps us ensure consistent model performance, which is vital for maintaining user satisfaction and trust.
The integration with APIs like OpenAI and Langchain makes it incredibly easy to use with our existing models. The ability to define tests in JSON or YAML formats is a game changer for our workflow.
One aspect I would like to see improved is the documentation. While it's generally helpful, some sections could be more detailed, especially for new users.
It allows us to automate the evaluation process, which significantly reduces the time spent on manual testing. This has increased our deployment speed and improved our model's reliability.
I love the versatility of BenchLLM. The ability to choose between automated, interactive, or custom evaluation strategies allows us to tailor the testing process to our specific needs. The quality reports generated are incredibly detailed and help us pinpoint areas for improvement.
The only downside I've encountered is that the command-line interface can be a bit daunting for newcomers. It took some time to get accustomed to the various commands and options available.
BenchLLM helps us identify performance regressions in our LLM applications effectively. This is crucial for maintaining high-quality outputs in our production environment, ultimately saving us time and resources on manual testing.
The ease of integrating it into our CI/CD pipeline has been a game changer for our team.
I would love to see more community support or forums where users can share tips and tricks.
It helps us ensure our models' performance remains at a high standard, which is crucial for maintaining client trust.
I appreciate the automated evaluation features. They save time and provide consistent results that we can rely on.
The setup can be a bit overwhelming with so many options available, but once configured, it works seamlessly.
It allows us to identify regressions quickly, which is crucial for maintaining the quality of our AI applications.
The command-line interface is intuitive for those familiar with CLI tools. It integrates well into our existing development workflows.
Occasionally, I find the error messages a bit cryptic, which can lead to confusion when troubleshooting.
BenchLLM helps us ensure our language models perform as expected before going into production, thus maintaining the integrity of our outputs.
I appreciate the clarity of the quality reports. They make it easy to communicate model performance to my team.
The CLI can be a bit daunting for beginners, and a GUI option would make it more accessible.
It helps in early detection of performance issues, which is essential for maintaining user trust in our AI applications.
The thoroughness of the evaluation process is impressive. It covers all aspects of model performance.
Occasionally, the interface can be a bit confusing, especially when trying to access advanced features.
It helps us identify and rectify issues in our models early on, which is crucial for maintaining high standards.
The performance monitoring capabilities are top-notch. They keep us informed about any regressions in real-time.
I would appreciate a more user-friendly guide for troubleshooting common issues.
It allows us to ensure consistent performance of our language models, which is essential for user satisfaction.
The comprehensive reporting features provide valuable insights into model performance, helping us improve continuously.
The command-line interface can be challenging for beginners, but it’s worth the effort to learn.
It ensures we can detect regressions early, which is vital for maintaining the quality of our AI applications.
The detailed quality reports and monitoring features make it a powerful tool in our testing suite.
The CLI can be overwhelming for first-time users, but once you get used to it, it's quite effective.
It helps us avoid potential issues in production by identifying regressions early in the deployment cycle.
I appreciate the flexibility in evaluation strategies. The ability to choose how we want to test our models helps us adapt to different project needs.
I found the initial setup a bit complex. It took a while to configure everything to work with our existing infrastructure.
BenchLLM allows us to ensure that our language models perform consistently. This is crucial for our applications, where even minor regressions can lead to significant user dissatisfaction.
The tool is robust and provides a comprehensive evaluation framework, which is essential for our development process.
It occasionally requires extensive configuration to set up tests, which can be time-consuming.
It addresses the challenge of ensuring consistent model performance, which is vital for our clients' trust and satisfaction.
The customization options for tests are incredibly useful, allowing us to adapt evaluations to specific use cases.
Sometimes the performance can lag during extensive evaluations, but overall, it’s manageable.
It ensures that we maintain high standards in model performance, which is critical for user satisfaction.
The ability to customize tests is fantastic. We can create tailored evaluations that suit our unique requirements.
The software can be a bit resource-intensive, especially during extensive evaluations.
It helps us catch issues early in the development process, reducing potential downtime after deployment.
The detailed reports are very helpful for our team when making improvements to our models.
I sometimes find the command-line interface a bit challenging, especially when I need to troubleshoot.
It allows us to monitor our models effectively, ensuring they perform well in production environments.
I love the detailed quality reports it generates. They provide in-depth insights into the performance of my models, making it easy to identify areas for improvement.
Sometimes, the command-line interface can be overwhelming for new users. A graphical interface would be great for those less comfortable with CLI.
BenchLLM helps me identify performance regressions in production environments quickly. This proactive monitoring allows me to maintain high-quality standards in my applications.
The reporting features are excellent; I can track model performance over time and identify trends.
The learning curve is a bit steep for new users, especially those unfamiliar with LLMs.
It helps streamline the testing process for our LLM applications, significantly reducing the time to deployment.
The ability to define tests in JSON or YAML formats is incredibly convenient and aligns well with our existing workflows.
It can take some time to get used to all the features, but it's worth it once you do.
It addresses the need for thorough evaluations of our AI models, helping us maintain high standards in quality.
The flexibility in evaluation strategies is fantastic! I can customize tests based on my specific requirements, which has significantly improved my workflow.
The initial setup process took some time to figure out, but once I got past that, everything was smooth sailing.
It assists me in evaluating various LLMs against APIs like OpenAI effectively. This has saved me time and resources in selecting the best model for my tasks.
The flexibility it offers in defining tests is impressive. We can customize evaluations to our specific use cases.
Sometimes the setup process can feel cumbersome, particularly for new users who are unfamiliar with CLI tools.
It allows us to effectively monitor model performance, which is essential for ensuring high-quality outputs.
The detailed quality reports are invaluable. They help us understand how our models are performing and where we can improve.
I found the documentation a bit lacking in certain areas, which can lead to confusion.
It allows us to proactively monitor our models, ensuring we deliver reliable outputs to our users.
The testing framework is extremely robust and highly customizable to suit our diverse project needs.
The documentation could use some improvement, especially for complex feature explanations.
It allows us to ensure that our models maintain high performance levels, crucial for user satisfaction.
The automated testing feature is a game changer. It allows me to run tests without manual intervention, which speeds up our CI/CD pipeline.
While the reports are detailed, they can sometimes be a bit too technical for stakeholders who are not familiar with AI model evaluations.
It helps me ensure that the models I deploy are performing optimally and not regressing over time, which is crucial for maintaining user satisfaction.
The ability to define tests in both JSON and YAML formats is incredibly flexible and user-friendly.
I would like to see more community resources and forums for user support.
It helps us maintain high-quality outputs in our applications by allowing comprehensive evaluations.
The customization options for test definitions are fantastic. We can align evaluations closely with our project goals.
The learning curve can be steep for those not familiar with command-line tools.
It helps us ensure our models are performing optimally, which is essential for delivering high-quality AI solutions.
The quality of the reports generated is excellent. They provide actionable insights that are easy to implement.
I would appreciate more examples in the documentation to help illustrate some of the more complex features.
It allows us to maintain high-quality outputs and quickly identify any issues that arise during model evaluations.
The flexibility in defining tests is excellent. We can tailor our evaluation to suit specific project requirements.
I wish there were more tutorials available to help new users get started quickly.
It helps us ensure our models are performing optimally, which is crucial for delivering high-quality AI solutions to our clients.
The ability to define tests in JSON or YAML is fantastic. It makes it easy to integrate with our existing workflows.
Sometimes, I wish the performance metrics were more comprehensive. Additional metrics would help in better evaluating model performance.
BenchLLM helps us maintain high standards by allowing for thorough testing of our AI applications, which is critical for user satisfaction.
The real-time performance monitoring is a game changer for our development process.
The initial configuration can be somewhat time-consuming, but the benefits outweigh this.
It allows us to catch regressions quickly, ensuring a smooth user experience in our applications.
The reporting features are top-notch, providing valuable insights that help us improve our models.
I found the CLI interface a bit challenging initially, but it becomes easier with practice.
It helps ensure that our AI applications deliver consistent and reliable results, which is crucial for user satisfaction.
I appreciate the depth of the quality reports. They provide insights that we can act on to improve our models.
The setup process can be quite involved, which might be intimidating for new users.
It helps us automate the evaluation process, ensuring we catch any issues before they affect our users.
The integration with APIs like OpenAI is seamless, allowing me to test various models efficiently.
Sometimes the documentation can be a bit sparse on certain advanced features.
It helps ensure that my models are not just functioning, but also performing at their best, which is crucial for our competitive edge.
The ease of integrating BenchLLM into our existing CI/CD pipelines has been remarkable. It fits perfectly into our workflow.
It would be great if there were more built-in templates for common testing scenarios to save time in configuration.
It allows me to monitor model performance continuously, which helps in quickly addressing any issues that arise post-deployment.
The custom evaluation strategies let me tailor tests specifically for my model requirements, which is incredibly helpful.
I wish there were more tutorials available for advanced features; it took me some time to explore everything.
It solves the problem of inconsistent model performance in production, allowing me to maintain high-quality outputs for my users.
The ability to run automated tests has really streamlined our development process. It integrates well with our CI/CD setup.
Sometimes the results can be overly technical for non-engineering stakeholders.
It helps maintain high standards in model performance, ensuring that our AI applications meet user expectations.
The user-friendly CLI is a major advantage. It fits seamlessly into our CI/CD pipeline, allowing us to monitor model performance continuously. The detailed quality reports are also a huge plus.
Sometimes, the test execution can be slow, especially with large datasets. I wish there was an option to speed up the process without compromising details.
It helps us maintain the quality and performance of our AI models. By detecting regressions early, we can address issues before they impact our users, which is vital in maintaining client trust.
The tool is incredibly efficient for automating evaluations. This has cut down on our testing time dramatically.
The learning curve for new users might be steep due to the variety of features and options available.
It helps us streamline our testing process, which has improved our overall workflow and productivity.